Blog
13.12.2024
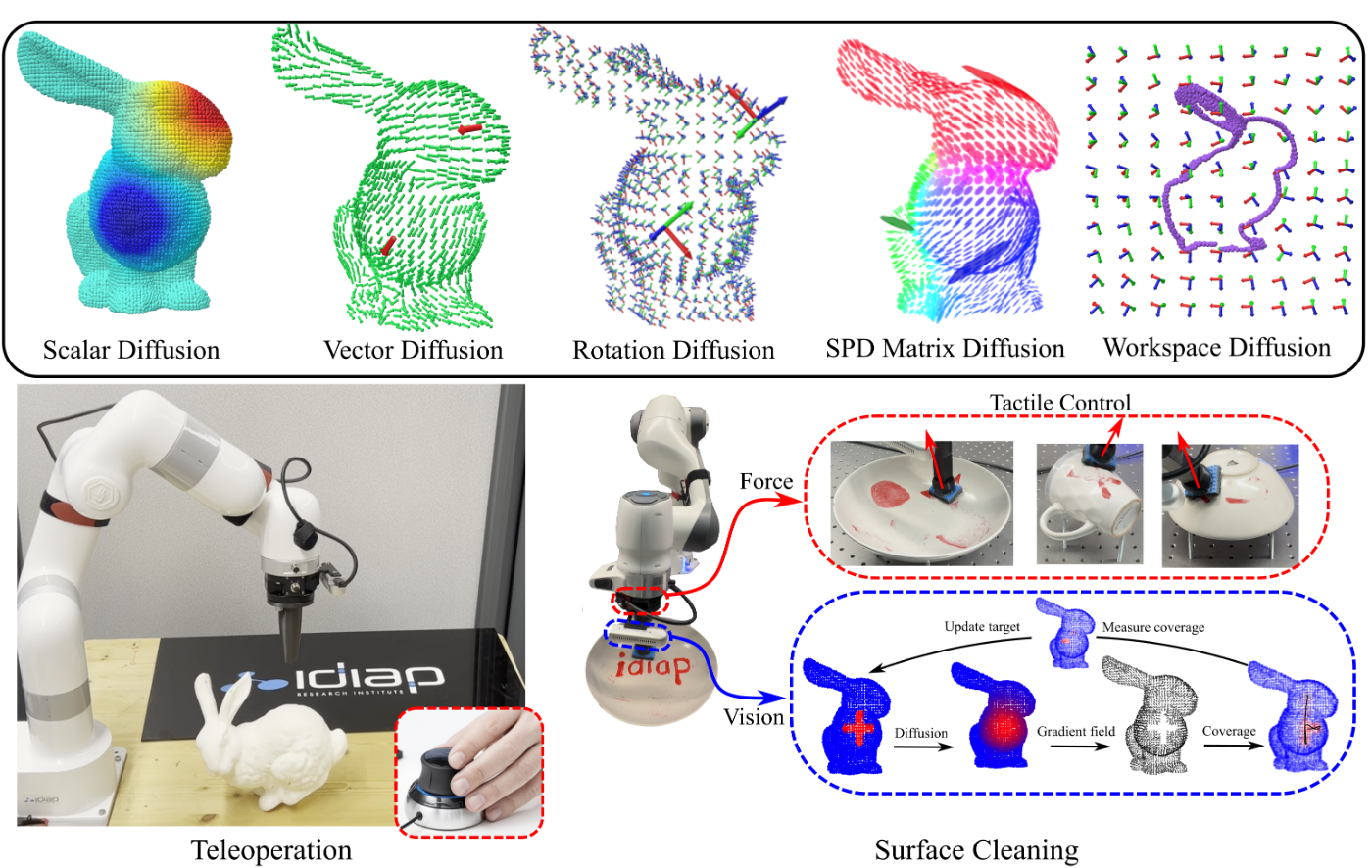
The Challenge of Curved Object Surfaces
A robot’s workspace often comprises diverse surfaces that may be partial, disconnected, or difficult to model. Some surfaces can be described using geometric primitives, such as planes or spheres, which might represent workspace boundaries. Others are derived from robot meshes or captured as point clouds during operation.
Surface Geometry as a Structure for Robotic Manipulation
Project partners from the Idiap Research Institute developed geometric representations to model the surfaces in the robot’s workspace, which can simplify a wide range of tasks, including surface cleaning, teleoperation, and object-centric learning, by leveraging diffusion principles. Diffusion fields smooth irregularities while preserving essential geometric features, enabling more intuitive and robust robotic interactions.
Validating Our Approach: IntelliMan Use Cases and Dissemination Activities
We tested our approach in two distinct applications inspired by Use Case 2: Manipulation in Daily-life Kitchen Activities and Use Case 4: Fresh Food Handling from the IntelliMan project:
- Cleaning on Curved Surfaces
- Object-Centric Teleoperation
These tests included presenting a video demonstration of cleaning on curved surfaces at ICRA@40 and a live demonstration of object-centric teleoperation at the Swiss Robotics Day 2024 in Basel.
Future Plans: Generalizing Manipulation with Object-Centric Diffusion Fields
Our future goal is to leverage object-centric diffusion fields for transfer learning tasks. Specifically, we aim to generalize a demonstrated manipulation task on one object to other similar objects, using diffused coordinate systems. The diffused coordinate systems form a smooth field across the workspace, locally encoding the key directions for interacting with objects. As a result, the local coordinate systems naturally serve as a basis for encoding demonstrations. Given that we can compute the diffused fields for a given object online using its point cloud, transferring a demonstrated task becomes a matter of replaying the demonstration in the new object’s diffused coordinate systems.
We plan to apply this methodology in the following scenarios:
- Demonstrating a task on one piece of kitchenware, and generalizing it to unseen but similar items. Use Case 2: Manipulation in Daily-life Kitchen Activities
- Modeling a pile of small fruits as a curved surface and generalizing the manipulation for efficient handling. Use Case 4: Fresh Food Handling